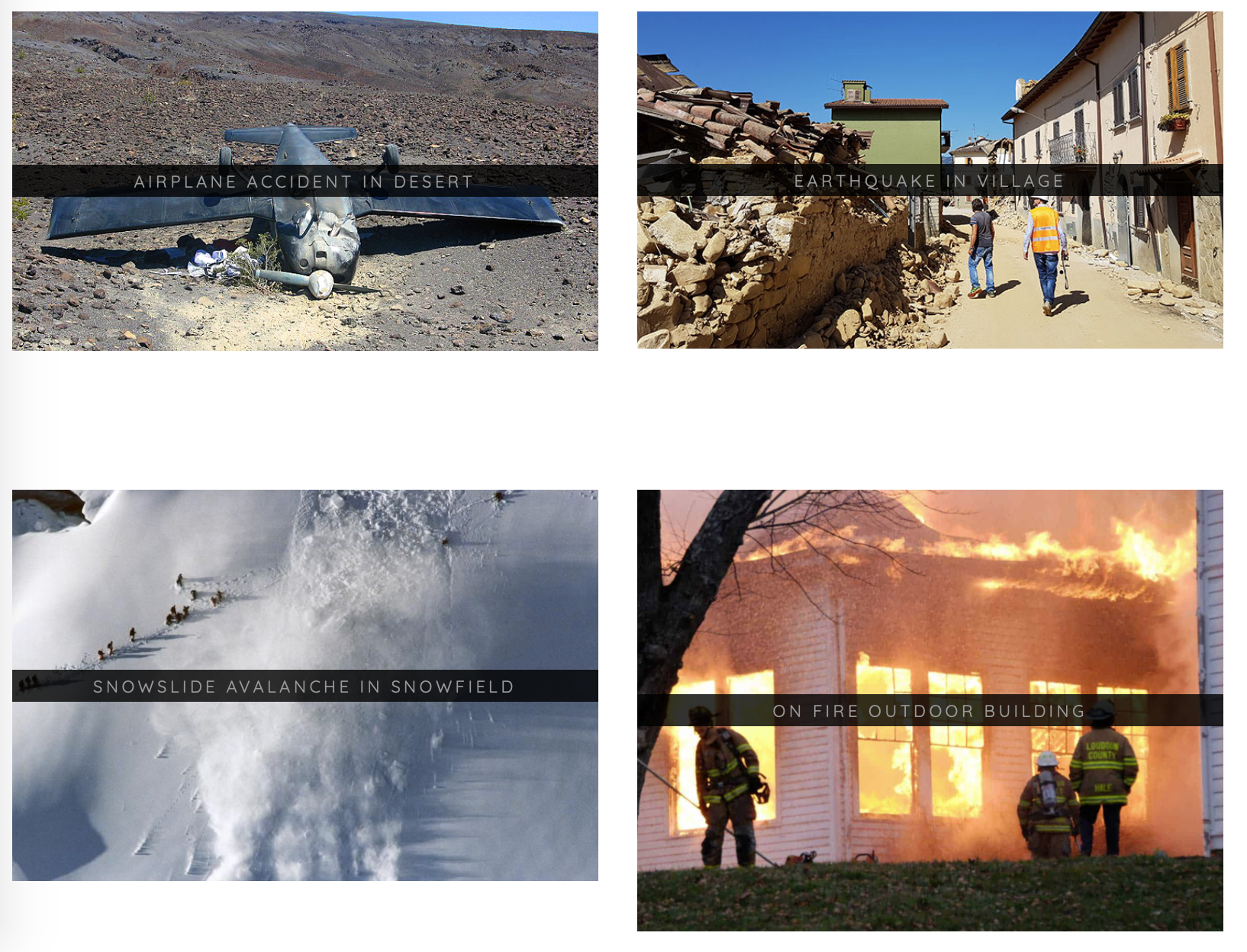
When a natural disaster occurs, on-the-ground emergency response teams act quickly to make life-saving decisions. Reducing the response time in such situations is critical to reduce damage impact and save lives. Helpful efforts are being taken to reduce the burden, such as a damage assessment tool by UNDP, though few automated methods exist. In recent work, MIT is creating tools that can automatically analyze images.
At the upcoming European Conference on Computer Vision (ECCV) conference, researchers from MIT and QCRI are presenting a new computer vision model capable of detecting incidents in images posted on social media platforms, such as Twitter and Flickr. They are also releasing the Incidents Dataset, a collection of 446,684 images labeled with 43 different incidents, including earthquakes, floods, wildfires, and car accidents. The paper, code, and data is available at the project page with interactive demos.
“I’m excited for this dataset to enable further research in detecting incidents in images, and to ideally spur interest in the computer vision community in general,” says Ethan Weber, an author on the paper and MEng student working with Professor Antonio Torralba.
The team’s new dataset aims to fill a void in the field, where existing datasets are limited in both the number of images and the diversity of incident categories. The authors go on to explain how the dataset was created, how a model can be created to detect incidents in images, and how to filter for incidents in noisy social media data.
One experiment filters 40 million Flickr images to find incidents. Additional experiments filter images posted on Twitter during earthquakes, floods, and other natural disasters. For example, the team filtered natural disaster-related tweets into specific incidents in a process that was validated by correlating tweet frequency with databases provided by the National Oceanic and Atmospheric Administration (NOAA).
As interest in automated response grows, competitions have emerged to further increase efforts in this direction–though not necessarily restricted to using images. The National Institute of Standards of Technology (NIST) launched a competition to promote fast analysis of unstructured data streams (social media, surveillance feeds, audio, text). Furthermore, last year the Defense Innovation Unit (DIU) hosted a challenge to advance building damage assessment techniques in satellite imagery. Instead of having humans look at satellite images and label where damage occurred, computers can help with rapid analysis.
Weber says that both social media and satellite imagery are valuable forms of data to help with emergency response. Social media provides on-the-ground insights, while satellite imagery provides expansive insights, such as determining which areas are most affected by a wildfire. Realizing this interconnectedness, Weber and MIT alum Hassan Kane teamed up and participated in the DIU challenge. Their team placed on the leaderboard for damage assessment, and they presented their award-winning work in the AI for Earth Science workshop at the ICLR 2020 conference.
With computer vision showing promising signs in both on-the-ground and satellite imagery, the MIT and QCRI researchers are now working on next steps. Enabled by the new ECCV dataset, the researchers hope to go beyond detecting incidents in damage.
“Now that we have the data, we’re interested in localizing and quantifying damage,” says Weber. “We’re working with emergency-response organizations to stay focussed and create research with real-world benefits.”
The Incidents Dataset presented in the ECCV paper is an effort by many contributors, including Weber, Nuria Marzo, Dim Papadopoulos, Aritro Biswas, Agata Lapedriza, Ferda Ofli, Muhammad Imran, and Antonio Torralba. More details on the study can be found on the project site.